Differentiable Photon Mapping using Generalized Path Gradients
Published in Siggraph Asia, 2024
Jiankai Xing, Zengyu Li, Fujun Luan, Kun Xu
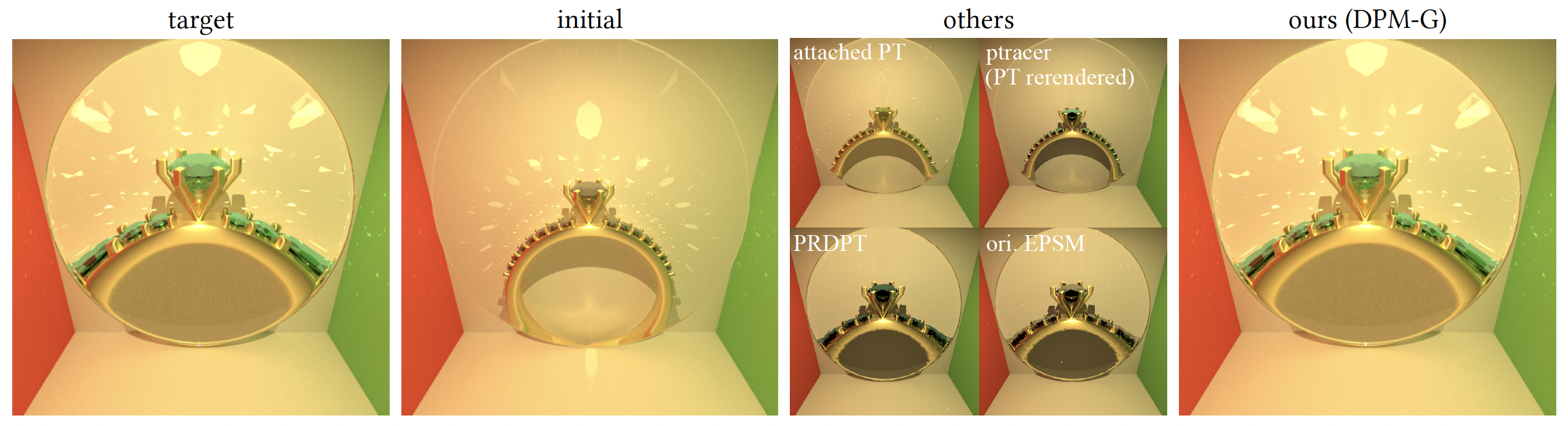
Abstract
Photon mapping is a fundamental and practical Monte Carlo rendering technique for efficiently simulating global illumination effects, especially for caustics and specular-diffuse-specular (SDS) paths. In this paper, we present the first differentiable rendering method for photon mapping. The core of our method is a newly introduced concept named generalized path gradients. Based on the extended path space manifolds (EPSMs) [Xing et al.2023], the generalized path gradients define the derivatives of the vertex positions and color contributions of a path with respect to scene parameters under given geometric constraints. By formalizing photon mapping as a path sampling technique through vertex merging [Georgiev et al. 2012] and incorporating a smooth differentiable density estimation kernel, we enable the differentiation of the photon mapping algorithms based on the theoretical results of generalized path gradients. Experiments demonstrate that our method is more effective than state-ofthe-art physics-based differentiable rendering methods in inverse rendering applications involving difficult illumination paths, especially SDS paths.
Recommended Bibtex Citation
@article{Xing2024DPMG,
title = {Differentiable Photon Mapping using Generalized Path Gradients},
author = {Xing, Jiankai and Li, Zengyu and Luan, Fujun and Xu, Kun},
year = {2024},
month = {dec},
url = {https://doi.org/10.1145/3687958},
articleno = {257},
numpages = {14},
journal = {ACM Trans. Graph.},
volume = {43},
number = {6},
publisher = {Association for Computing Machinery},
}